Are Insurers Leveraging Bots to Boost Workforce Productivity?
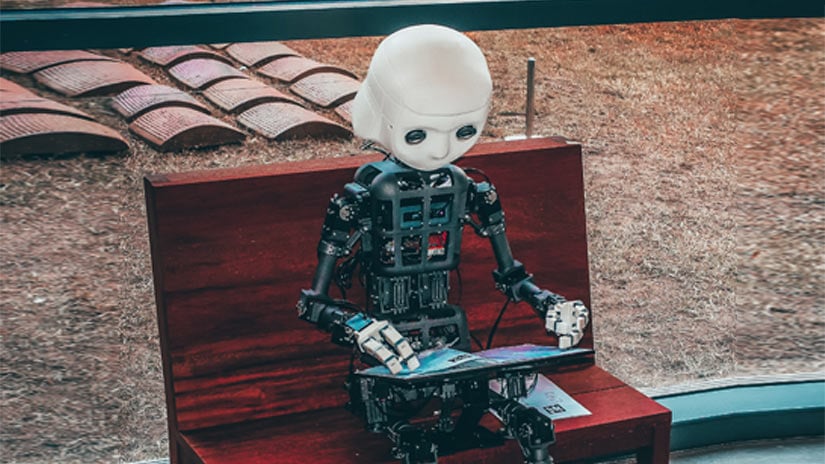
Not all bots are chatbots. A bot is a computer application designed to automatically perform repetitive or logic-based tasks and a chatbot is the same thing but with the focus as simulated conversation. Bots are not a new concept, even though the benefits of chatbots in insurance is gaining more acceptance in the last few years. Search engines like Google were the early movers, using bots to analyze content and index the web - a feat that would be impossible for humans to accomplish.
Insurance bots hold the promise of easing the complexity of insurance transactions. Over the next decade, bot technology (including chatbots) will play a bigger role across the insurance value chain.
The different ways Insurers are using bots
Geico uses a mobile virtual assistant “Kate” to provide answers to policy coverage and billing questions. Lemonade Insurance Company has ‘Maya’, their insurance chatbot that uses plain English and brought 70,000 customers online in their first year, with no human agents stepping in during the initial process. These and others are examples of user-facing, superstar insurance chatbots. However, there are other innovative bots working quietly behind the scene, not getting the publicity that the chatbots do.
Many insurance carriers begin their chatbot integration with customer service use cases but sales is another great area to utilize these bots. Bots can guide a customer through the quotation process, answering all their questions to get a positive outcome and then hand-off to a human agent if need be. There are other ways too that insurance bots are adding value to workflows.
Insurance companies are using AI bots in myriad ways, the most popular are:
-
Directly helping customers with personalized quotes or indirectly helping human agents to offer better quotes
-
Manage claims quickly and efficiently
-
Simplify regular tasks like account updates and payments
-
Offer relevant advice on coverage
Antony Xavier, co-founder SImpleSolve, observes “We’re seeing many insurers asking us about bots but they don’t necessarily know how the technology can be applied in the insurance value chain”.
SimpleINSPIRES’s bot, Tara, comes with two-way integration with any application and can be custom trained specifically to a customer and their policy products. Tara is a Smart BOT and supports intelligent underwriting in a way that was not possible before. It is the foundation and user interface for Machine Learning and AI-driven underwriting & workflow. For instance, Tara can prompt agents in real-time about high-risk concentration just as they are ready to provide a quote to a new customer or even alert that the NB they are quoting was a prior insured with claims.
If you liked this, also read: How Tech Improves Efficiencies in Business Process Management
These are perfect points to segue into an explanation of the different bot technologies and their corresponding capabilities
The technology behind the bots
Bot applications are evolving rapidly thanks to emerging technologies such as NLP and AI that are expanding bot capabilities. Two commonly used technologies power most bots.
-
Rule-based bots using Natural Language Processing
Rule-based bots work on a predefined set of questions and use an if/then logic. This makes them easier to train and reduces implementation costs.
Like a flowchart, the logic works on mapping out conversations that a customer might ask and how the chatbot can respond. These rules can have varying complexity and depend on a fixed script. They can be integrated with Natural Language Understanding (NLU) engines that help to better understand and respond to a broader range of language variations.
Since these bots can only be trained and cannot self-learn, they must hand over to a human agent if they cannot find an answer in the database. They can also only work in the scenarios they are trained for. Though the bots can be embedded with additional data as new conversational patterns arise from customer interactions, this requires scheduled logic updates at an additional financial cost.
Since rule-based bots are less costly and easier to build, they are best suited for companies that have a specific goal they want to lead their users to or if the chatbot should funnel users to human agents.
2. Conversation AI bots
AI-enabled Smart Chatbots are the future of chatbots in insurance. They are the very opposite of rule-based bots since conversational AI is free-flowing and understands intent as well as sentiment in language. Smart bots are designed for near-human interactions and rely on Natural Language Processing, Natural Language Understanding, Machine Learning, Deep Learning, and Predictive Analytics.
Since Smart Bots learn from patterns and previous experience they need a huge amount of training data as well as highly-trained specialists. This can be a limiting factor for insurance carriers that do not have a copious amount of historical organized data.
The standard architecture in these conversational AI systems require different modules to address a single query
-
Automatic speech recognizer (ASR) - This takes raw audio or text and converts it to a hypothesis.
-
Natural language understanding module (NLU) - This module receives the hypothesis and understands the user’s intent and converts it into semantic slots.
-
A dialog manager (DM)- The DM generally controls the dialog flow by interpreting the inputs from ASR and NLU. It can also interact with external knowledge sources, producing the final output to the user.
-
Natural language generator (NLG) - AI composes an appropriate response using NLG
-
Text-to-speech synthesizer - The last stage in the conversation system takes the NLG response text and converts it into natural-sounding speech. The vocal clarity is achieved using deep neural networks that create a near-human intonation (think Siri or even your GPS).
Conversation AI bots tap into various systems such as websites, databases, and APIs. If any of these resources are updated, the changes are automatically applied to the bot interface. While a lot goes into setting up the insurance AI bot systems, once up, AI systems have the self-learning elasticity that rule-based bots don’t. This makes Insurance AI bots more cost-efficient in the long run compared to rule-based bots that require continuous maintenance to stay relevant.
Insurance bots can often access sensitive information such as personal identifiable information (PII). AI bots have the ability to change communication from voice to text and also redirect customers to a secure link at any point in the conversation,
Ideally, automation in insurance should address processes that are a bottleneck or take too much human effort.
Human bias can unconsciously affect AI systems
There is, though, a cautionary note that must be highlighted here with regard to training data sets. Data is used to train AI models and if that data is not broad enough or the scenarios are too limited then the AI system is able to generalize only on the data it has. For example, Amazon stopped using a hiring algorithm because it was found to discriminately favor male applicants. It had a loaded score for words like “executed” and “captured” that were found to be more popularly used in male resumes. While such situations can never be completely avoided, insurers and their insurtech partners must put in place ways to regulate against its occurrence.
Topics: AI Chat Assistant


