Gen AI's Big Wins in Insurance But What About The Risks?
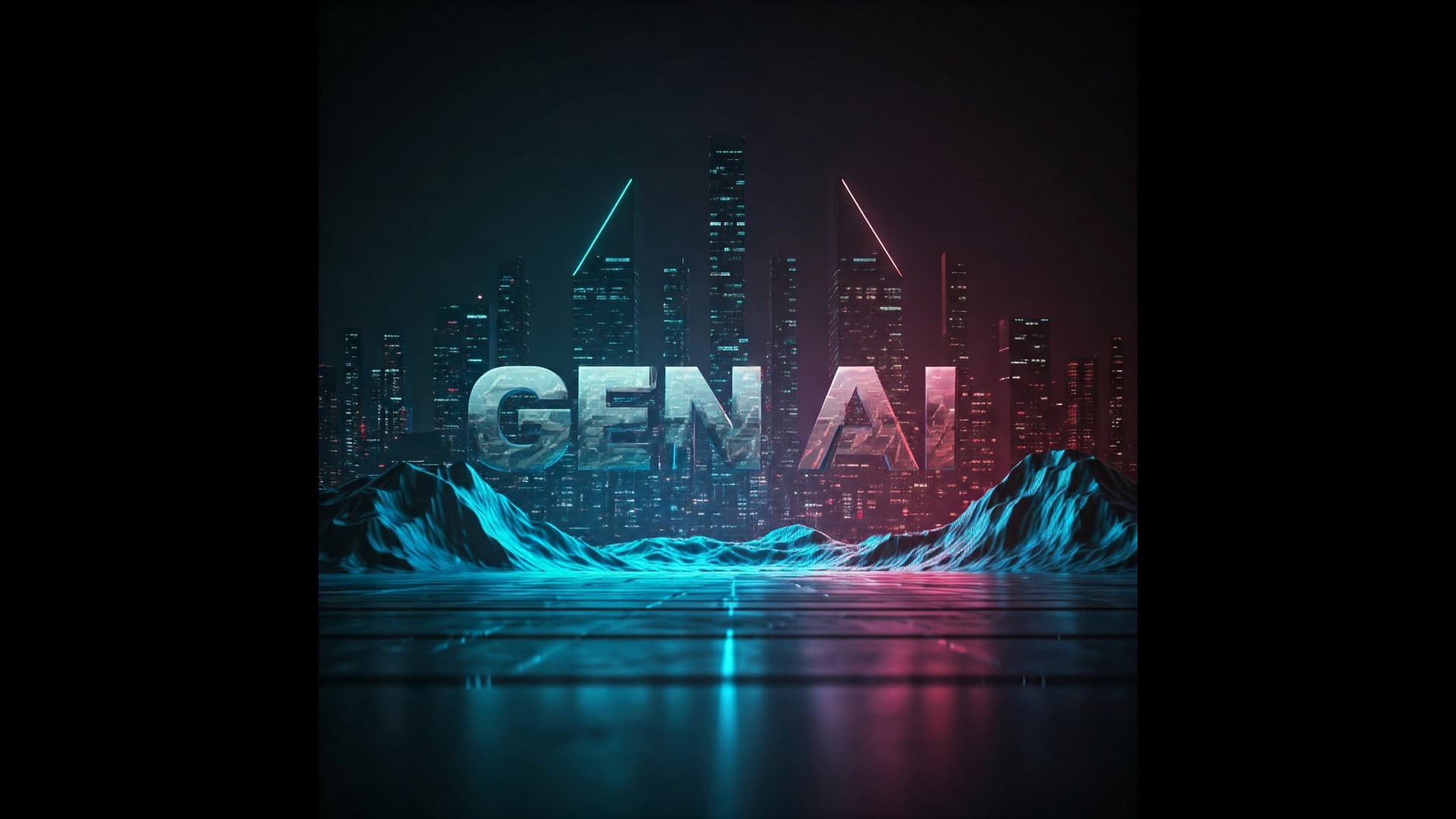
The property and casualty sector flipped a USD 8.5 billion underwriting loss in Q1 2023 into a USD 9.3 billion gain in Q1 2024, with a combined ratio of 94.2% - figures below 100% indicate an underwriting profit. But in a market that refuses to stand still, staying ahead will require insurers to rethink their tech game and push the boundaries of innovation.
Three out of four U.S. insurers already leverage Generative AI (Gen AI) in at least one business area, with claims processing and customer service leading the way (Deloitte survey of 200 insurance executives). However, scaling Generative AI insurance adoption isn't straightforward. Data security, privacy issues, and integration challenges remain top concerns for insurers wanting to expand AI across their organizations.
The opportunities are immense, but so are the risks. There are compliance hurdles and operational vulnerabilities to be navigated to fully harness AI's potential without jeopardizing profitability or governance.
Gen AI in Insurance - Real Transformation in Operations
According to the Capgemini Research Institute Report for 2025, 67% of top-performing insurers are prepared to use Generative AI to enhance policyholder experiences and streamline operations
Generative AI differs from traditional AI in that it creates new data and content rather than just analyzing existing data or automating predefined tasks. Here are some concrete instances illustrating its impact:
Gen AI Automates Policy Document Generation & Enhances Claims Efficiency
One of the most effective Gen AI in insurance use cases is automating the creation of policy documents. By inputting customer-specific data, the AI generates tailored policy documents that meet regulatory standards and customer needs, significantly reducing the time and manual effort previously required.
Additionally, Gen AI can be deployed alongside insurance industry knowledge workers, such as underwriters, actuaries, claims adjusters, and engineers, to heighten productivity and efficiency.
The technology helps summarize and synthesize large volumes of content gathered throughout the claims lifecycle, including call transcripts, notes, and legal and medical paperwork, which is particularly useful in property and casualty insurance. This allows companies to compress the claims lifecycle dramatically, improving speed and accuracy while freeing up valuable resources.
Synthetic Data for Model Training
Generative AI is shaking things up by letting insurers simulate different risk scenarios using past data. It’s like having a crystal ball, but backed by real numbers. These datasets mimic real customer data, allowing for robust training of machine learning models in areas like fraud detection and risk assessment.
By analyzing previous customer data, insurance generative AI can create realistic simulations of potential future risks. These simulations aren’t just for show – they help train predictive models, making it easier to fine-tune risk estimates and set premiums more accurately. It’s a smarter, faster way to stay ahead of the curve.
Gen AI Helps in Personalized Marketing Content
Insurers are tapping into Gen AI to craft marketing that feels personal and hits the right notes with each client. By digging into customer data and preferences, Gen AI can quickly turn out tailored brochures, blog posts, social media content, and emails that speak directly to different user segments. The result? More engagement and better conversion rates. Almost every marketing team has Gen AI in their marketing toolbox anyway, upping the ante on automation is just a step further.
It doesn’t stop at marketing. GenAI also steps in to handle direct customer interactions – drafting service emails, policy updates, and automated messages. This keeps communication timely and relevant throughout the customer journey, making clients feel heard and boosting overall satisfaction and loyalty. A caveat here, it is preferable to have a human in the final review.
Enhanced Customer Interactions
Some insurers have integrated Gen AI into their customer service platforms to create more natural, context-aware conversations. Gen AI analyzes past interactions and policy details to provide hyper-personalized responses to customer inquiries. For example, when a policyholder asks about claim status or coverage specifics, the AI responds with precise information pulled from internal systems, reducing the need for human intervention and significantly cutting response times.
These examples demonstrate how insurance Gen AI use cases are not merely an extension of traditional AI but a catalyst for innovative solutions, driving efficiency and enhancing customer experiences.
Navigating the Risks Associated with Gen AI
Generative AI, as the name suggests, generates new content by learning from data inputs and powerhousing AI’s ability to produce text, reports, and insights that mirror human output. This makes it a powerful tool for enhancing customer experiences and optimizing operations but its integration also presents complex risks and compliance challenges that demand expert attention.
Model Hallucinations and Decision Integrity
Generative AI models can produce outputs that appear plausible but are factually incorrect, known as "hallucinations." In insurance, such inaccuracies can lead to flawed risk assessments, inappropriate policy pricing, and erroneous claims decisions, undermining the integrity of underwriting and claims processes.
Despite the risk of hallucinations, Gen AI remains valuable in insurance because it boosts speed and efficiency, quickly generating drafts for a large number of clients or new coverage options. It ensures consistency by using standardized language across policies, reducing errors.
To avoid the pitfalls of Gen AI hallucinations, insurers are building safety nets – like validation checks and always keeping humans in the loop – to make sure AI-generated content is accurate and trustworthy.
Adversarial Attacks and System Vulnerabilities
Generative AI systems can be vulnerable to adversarial attacks, where bad actors feed in malicious inputs to trick the AI into making wrong decisions. In insurance, this could mean AI models being manipulated to approve fraudulent claims or alter risk assessments.
To protect against these risks, insurers should implement strong security measures like data encryption, secure model training practices, adversarial testing, and regular audits. Additionally, using robust authentication methods and ensuring continuous monitoring of AI systems for unusual behavior are essential for safeguarding against vulnerabilities.
Regulatory Compliance and Explainability
Regulators are stepping up their scrutiny of AI, particularly with the increasing popularity of Gen AI in the insurance sector. In the U.S., Colorado is leading efforts to establish a framework aimed at minimizing bias and discrimination in AI models applied to underwriting and claims processing.
The opacity of generative AI models, often referred to as "black boxes," poses challenges in regulatory compliance with the increasing requirement for explainability in decision-making processes. Insurers must ensure that AI-driven decisions are transparent and interpretable to meet regulatory standards and maintain customer trust. Adopting explainable AI (XAI) techniques can enhance transparency and facilitate compliance with legal and ethical standards.
In scenarios like USAA's AI-powered claims processing or Allstate's virtual adjusters, XAI ensures that the system's decisions—such as determining the severity of damage or prioritizing claims—are not black boxes. Instead, these systems generate detailed, understandable explanations for their actions, allowing insurers to confidently communicate decisions to policyholders and regulators.
Ethical Considerations and Bias Mitigation
Generative AI models, when trained on historical insurance data, can inadvertently learn and reproduce existing biases found in the data. For instance, if historical data reflects biased decision-making—such as offering lower coverage or higher premiums to certain demographic groups—AI models may perpetuate these biases, leading to unfair treatment of those groups.
To address this, insurers must apply targeted strategies such as retraining models on more diverse, representative datasets and implementing bias detection algorithms to identify skewed patterns. For example, they can use fairness-aware machine learning techniques that monitor and adjust decision-making processes in real time to ensure that the AI is not unfairly disadvantaging any protected group. Additionally, insurers can regularly audit AI outcomes and use human oversight to review decisions, ensuring they align with fairness standards.
Operational Risks and Governance
The integration of generative AI into insurance operations introduces risks related to system failures, data inaccuracies, and process disruptions. Establishing robust governance frameworks, including clear policies, accountability structures, and risk management protocols, is essential to manage these operational risks effectively. Regular audits and compliance checks can further ensure that AI systems operate within established guidelines and contribute positively to organizational objectives.
To drive meaningful business results with Gen AI, a clear strategy and collaboration across cross-disciplinary teams are essential. Gen AI’s insurance potential is best realized when integrated across various functions, requiring input from IT specialists, business leaders, and domain experts. Given the rapid evolution of Gen AI, organizations should avoid going it alone and instead seek partnerships and external expertise to navigate its complexities.
Topics: A.I. in Insurance


