The Business Drivers Behind Intelligent Underwriting in Risk Assessment
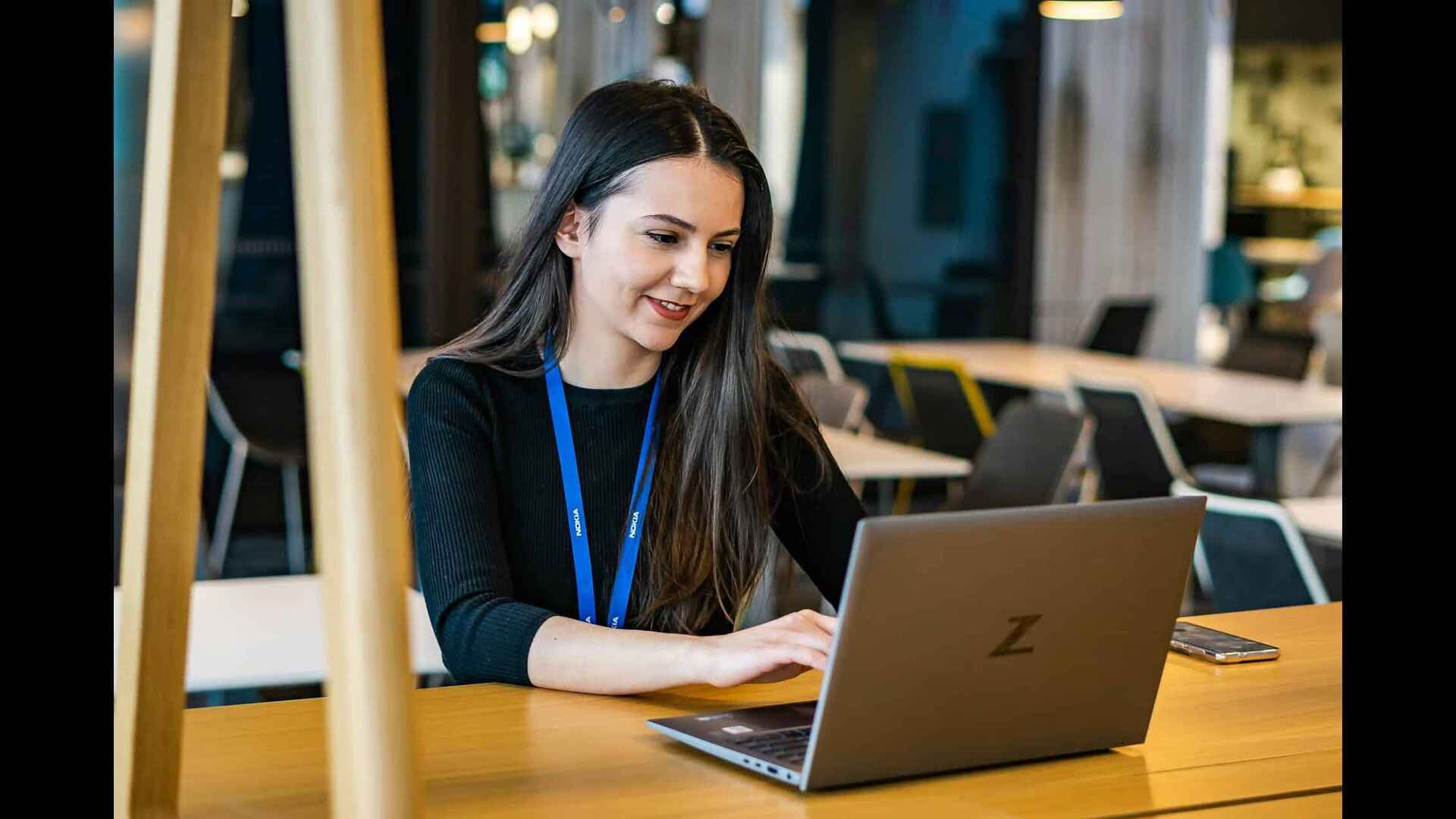
The insurance industry has always faced a delicate balancing act—delivering accurate risk assessments without sacrificing operational efficiency or profitability. Traditionally, underwriters have relied on siloed historical data, manual processes, and static models, often leading to high operational costs, inconsistent risk evaluations, and poor decision-making in complex scenarios.
Capgemini's latest report throws up some tough truths. Around 77% of insurers admit they’re struggling with incomplete risk evaluations. This is a major issue—without a solid data foundation, risk assessments are often way off the mark, impacting everything from pricing accuracy to policyholder trust. It’s like trying to hit a moving target while blindfolded.
Because of this, 73% of firms say their pricing accuracy is way off. If your pricing is shaky, it affects everything downstream—especially claims coverage. It’s not just about losing money on claims; inadequate pricing could eventually threaten an insurer’s solvency if the gaps persist. And that’s a risk no insurer wants to take.
The Business Drivers for Change—and the Tech Fueling It
Insurers have several strategic business drivers at the top of their minds as they evaluate their underwriting models and operations. Today’s intelligent underwriting technologies aren’t just enhancing efficiency—they’re reshaping how insurers approach risk, decisions, and profitability.
When we talk about the tech driving this transformation, we’re diving into the layered architecture of AI underwriting models, advanced machine learning techniques, and data integration systems that elevate underwriting precision and adaptability. Insurers using these tools are gaining advantages far beyond traditional methods, giving them an edge in a competitive market.
This change in underwriting techniques is because of these standout technologies:
1. AI-Driven Risk Models: Getting Precision Right
Intelligent underwriting relies on machine learning models that enhance risk prediction. Unlike traditional regression models, insurers are now using ensemble model techniques like Random Forests algorithms and Gradient Boosting Machines (GBMs) to harness real-time inputs from diverse sources such as IoT data, geospatial analytics, and customer behaviors. This dynamic input enables a more precise calculation of risk profiles, fine-tuning the underwriting process in ways that static models just can’t match.
-
Random Forests: This ensemble learning method builds multiple decision trees and merges them to improve the accuracy and stability of predictions. Combining multiple decision trees makes for better prediction accuracy. Random Forest algorithms make them ideal for assessing complex risk profiles, reducing overfitting, and supporting both risk classification and premium pricing across diverse insurance types.
-
Gradient Boosting Machines (GBMs): GBMs build models sequentially, with each new tree correcting the errors made by the previous ones. This method is highly flexible and can model complex nonlinear relationships, which is valuable in assessing diverse risk factors in underwriting. GBMs offer a well-rounded view of risk, a big advantage for high-risk areas like cyber insurance and natural catastrophe coverage.
A P&C insurer recently integrated GBMs to improve risk stratification for small business policies. By layering in data from third-party sources like financial stability reports and location-based risk indicators, they could tailor premiums more accurately. This approach not only sped up the underwriting process but allowed the insurer to offer more competitive premiums, positioning them ahead of competitors relying on traditional models.
2. Data Integration and Preprocessing: Speed Meets Accuracy
Optimizing risk assessment goes beyond model selection— it requires the integration of real-time data with legacy systems. Advanced data integration solutions capture, clean, and analyze disparate data sets with minimal manual intervention.
A growing number of P&C insurers are achieving substantial reductions in data integration time by using pre-built connectors and automated pipelines that significantly accelerate the process. For instance, some insurers report integration time savings of up to 80% by leveraging systems that automate connections to claims histories, risk data, and customer engagement records. This speed not only boosts operational efficiency but also enhances underwriting accuracy by keeping data current and relevant for decision-making.
Most P&C insurance executives (83%) agree that predictive models are going to be a game-changer for underwriting. But here's the catch—only 27% of them feel their companies have the advanced tools needed to make it happen.
So while the vision is there, the capabilities aren’t catching up yet. The real breakthrough starts with building a secure data ecosystem (Capgemini’s World Property and Casualty Insurance Report 2024).
3. Explainable AI (XAI): Transparency for Better Compliance and Trust
As AI becomes a bigger part of underwriting, transparency is crucial for regulatory compliance and internal audits. Here’s where Explainable AI (XAI) tools come in. With techniques like SHAP (SHapley Additive exPlanations) and LIME (Local Interpretable Model-Agnostic Explanations), insurers can trace how each variable influences underwriting decisions.
SHAP and LIME are open-source tools commonly used for machine learning model interpretation across various industries, including insurance. They offer insurers insights into how each feature or variable affects underwriting decisions, regardless of the specific model or platform in use.
SHAP, based on Shapley values from cooperative game theory, quantifies the contribution of each variable to the model’s outcome, while LIME explains individual predictions by approximating the model locally. These techniques help insurers maintain transparency and traceability in decision-making, which is valuable for regulatory compliance and enhancing stakeholder confidence.
Take an insurer focusing on cyber liability policies. By using SHAP to explain model decisions, underwriters could see how factors like network security scores or past incidents impacted premium pricing. Not only does this transparency aid in compliance, but it also strengthens trust with clients who can see why they’re rated a certain way.
4. Advanced Simulation Techniques: Anticipating High-Risk Scenarios
AI underwriting technology isn’t just about assessing risk; it’s also about anticipating it. Monte Carlo simulations and stochastic modeling are helping insurers run thousands of potential scenarios, equipping them to set premiums accurately for volatile risks. Markov chains, for example, are used to model probabilities in dynamic systems, like the likelihood of different risk transitions. This method helps insurers predict shifts in risk states over time, assisting in more accurate pricing and loss forecasting for complex products.
A practical application can be seen in catastrophe insurance. An insurer using Monte Carlo simulations for earthquake coverage could simulate multiple scenarios based on geological and historical data, resulting in a more precise pricing model. The result? Better financial stability for the insurer and more confidence for policyholders.
5. Real-Time Analytics and Continuous Underwriting: Insight-driven strategies
Perhaps one of the most profound shifts driven by intelligent underwriting solutions is the move towards continuous underwriting. Traditional models were static, with risk assessments done at the policy’s inception and often not revisited until renewal. However, with the advent of event-driven architectures, insurers can now process real-time data and adjust underwriting decisions dynamically.
Using technologies like Apache Kafka for real-time data streaming, insurers can continuously monitor changes in risk factors, such as fluctuating cyber threat levels or regional environmental risks, and update pricing or policy terms accordingly. This shift towards continuous risk monitoring is crucial for industries like cyber insurance, where threats evolve daily, and outdated static underwriting models expose insurers to significant risk
In the End, It’s All About Smarter Pricing
It all boils down to better pricing capabilities of intelligent underwriting techniques. By analyzing a business’s past claims and rate history and comparing it wiith the similar insured block for competitors in the same industry, it allows insurers to fine-tune quotes, aligning premiums more accurately with the actual risk profile.
Insurance firms embracing these technologies are witnessing gains across the board—from more competitive premium rates to improved operational efficiency and customer satisfaction. By going beyond the basics of AI and embracing integrated, real-time data analysis and sophisticated modeling techniques, these insurers aren’t just keeping pace; they’re setting the standard for a data-driven future in underwriting.
Topics: Risk Management


