Are Insurers Fully Tapping Into the Hidden Potential of Their Historical Data?
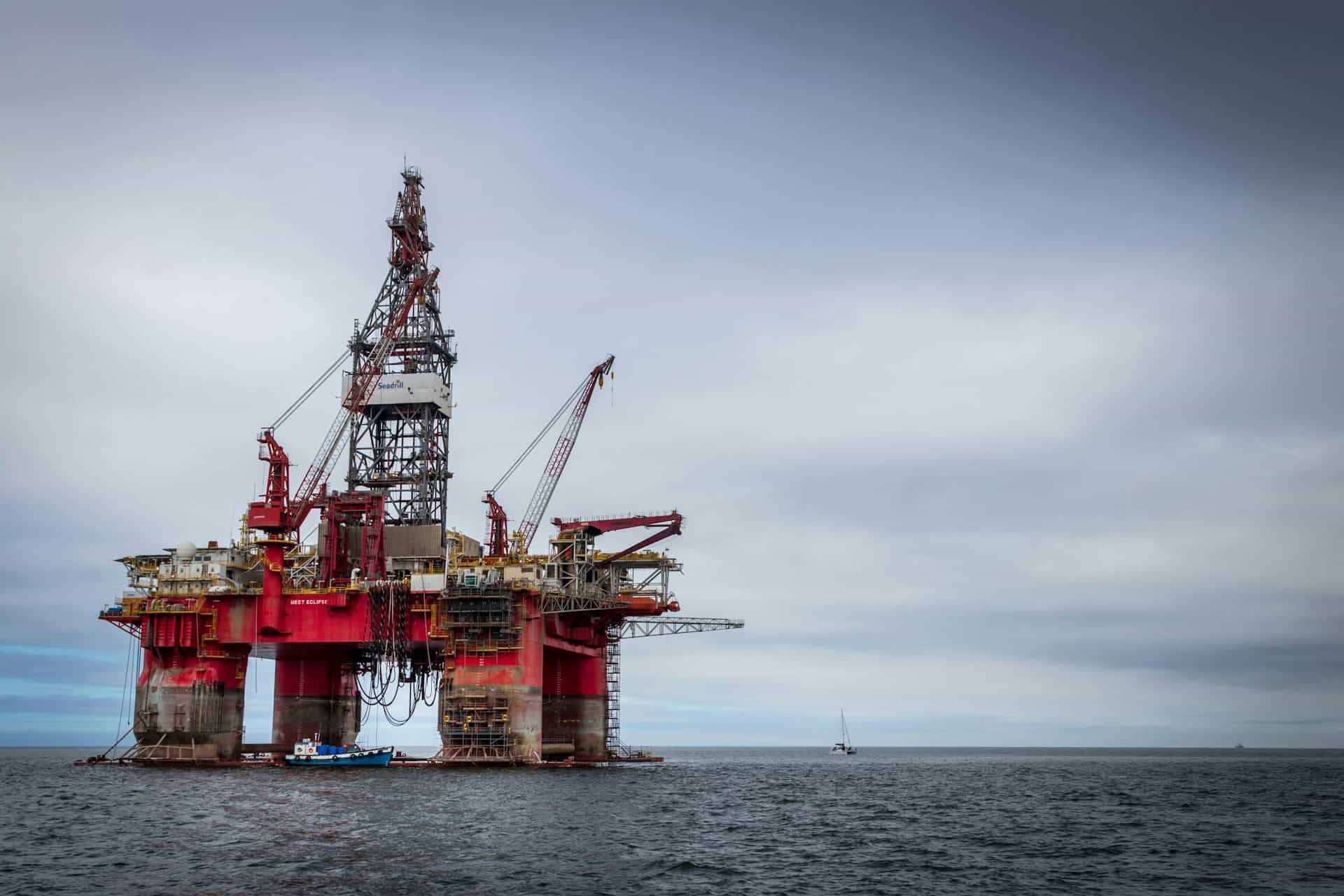
Data has always been the lifeblood of the insurance industry, driving decisions, pricing, and risk management. Insurers are sitting on a goldmine of historical data—rich in insights that could transform their strategies. Much of the value of their historical data remains untapped, trapped within models that no longer meet the industry's current demands. These models typically focus on linear projections based on past trends, which can fall short in today’s rapidly changing environment.
Insurance carriers are missing the opportunities to leverage advanced analytics, machine learning, and real-time data processing, which could reveal deeper insights and allow for more effective responses to emerging risks. Their technology platforms may not be helping either.
Research shows up to 74% of insurance companies depend on legacy systems for core functions. Old Tech has become the new risk. (Clearwater Analytics)
Are You Getting Critical Insights into Your Data?
As competition in the insurance industry intensifies, customer loyalty has seen a decline over the last 5 to 10 years. Insurers are now facing the challenge of not just retaining customers but also identifying and targeting the right ones. Historical data holds a treasure trove of information about customer behavior, risk patterns, and policy performance.
The seismic technological shifts in the last few years have changed our expectations of businesses and more so in insurance. It has created new types of risks and customers, forcing insurers to rethink and innovate their product offerings to stay competitive.
By not fully tapping into advanced data analytical models, insurers risk missing critical insights to enhance customer segmentation, improve acquisition strategies, and ultimately drive better business outcomes.
Think about how smart home gadgets are shaking up homeowners' insurance. As more Americans are buying smart thermostats, security cameras, and leak detectors, insurers are rethinking how they assess risk and price their policies competitively.
Take American Family Insurance, for example. They’re offering discounts to homeowners who install these smart devices. Why? Because these gadgets can help prevent costly issues like fires, burglaries, or water damage. So, instead of just sticking to the old ways of calculating premiums, insurers will need to adapt their products to fit this new tech-driven reality.
Regulatory Compliance: A Major Barrier to Effective Data Utilization
Regulatory compliance is a significant hurdle for insurers trying to harness the full potential of their historical data. The complexity of regulations surrounding insurance data privacy, security, and usage can severely limit how insurers leverage their vast data reserves. Regulations such as the California Consumer Privacy Act (CCPA) in America. impose strict controls on how data can be collected, stored, and used.
The Compliance Bottleneck
Since the mid-2000s, insurance businesses have increasingly required approval from their compliance departments before launching customer-related campaigns. These departments have to challenge their organizations from the compliance standpoint to lower potential risks to their company. This is only fair but this causes incumbents to stay put in the fear of overstepping regulatory boundaries.
Data Silos and Fragmentation
Most data-based regulations mandate carriers to furnish specific data, yet insurers often struggle to channel the correct information because it’s buried deep within vast repositories of documents scattered across different departments. Compliance requirements can worsen this issue by restricting data sharing both across borders and within different parts of the same organization.
This is where AI's role becomes crucial. AI-driven data management tools can sift through massive volumes of documents, extracting and organizing relevant data with speed and precision that manual processes can't match. By automating data retrieval and ensuring that the right information is readily available for compliance purposes, AI helps to alleviate the bottlenecks that can arise from fragmented and poorly managed data systems. This not only streamlines compliance efforts but also frees up resources that can be redirected toward more strategic initiatives.
According to a survey by McKinsey, 40% of insurance companies say that they struggle to leverage data effectively, and one of the main reasons is the problem of data silos.
Operational Costs and Resource Allocation
Ensuring compliance is not just a technical challenge but also a financial one. The costs associated with maintaining compliance—ranging from legal consultations to upgrading IT systems—are significant. A National Bureau of Economic Research working paper reports that average regulatory spending for US companies accounts for 1.34 percent of total wage costs. For insurance companies, it can range between 4-7% (Deloitte). This financial burden often diverts resources from innovation and growth initiatives, creating a balancing act between compliance and competitive advantage.
While simplified governance might still be a utopia, insurance has innovated considerably despite these challenges in the past few years. Modern insurance platforms have played their part in this transformation.
How Modern Insurance Platforms Help in Better Managing Data
Data isn't just an asset for insurers anymore—it's the backbone of their competitive edge. Modern insurance platforms go beyond basic management; they reshape how insurers leverage their mountains of data to thrive in a fast-evolving market.
Automated Compliance Management: Modern insurance platforms come equipped with automated compliance management tools that help insurers navigate the regulatory maze more efficiently. These platforms integrate data governance features that ensure insurance big data is handled according to the latest regulations, minimizing the risk of non-compliance. For instance, these platforms can automatically anonymize data or restrict access based on regulatory requirements, reducing manual oversight and errors.
Advanced Data Integration and Analytics: These platforms also facilitate the integration of data from disparate sources, breaking down silos while maintaining compliance. Cloud-based platforms like SimpleINSPIRE offer secure environments where data can be stored and processed across borders while adhering to local regulations.
The use of AI and machine learning within these platforms enables insurers to conduct more sophisticated analyses while staying compliant. AI can identify patterns in anonymized data that might not be evident with traditional methods, unlocking new insights without breaching regulatory constraints.
Enhanced Data Governance and Security: Robust data governance frameworks built into modern platforms ensure that data is not only compliant but also accurate and reliable. These platforms offer advanced tools for data lineage, quality control, and real-time auditing, which are essential for maintaining regulatory compliance. By ensuring that their insurance data is managed according to best practices, insurers can reduce the risk of regulatory breaches and the associated financial penalties.
Traditional Data Models vs Modern Analytical Models
Historically, insurers relied on static, often siloed datasets that were manually updated and used for retrospective analysis. Their predictive capabilities were limited to rule-based systems or basic statistical methods, which were rigid and maintained with predefined parameters. This made them less adaptable to new or unforeseen risks. Due to the limited variety in data and the inflexibility of these models, traditional analytics often led to conservative decision-making, which might not adequately account for emerging risks or changing market conditions.
In 2021, insurers invested $3.6 billion into big data analytics, leading to immediate gains like a 30% increase in efficiency and 60% better fraud detection. Today, these early investments are driving more personalized policy offerings, streamlined underwriting, and predictive fraud prevention
Modern models leverage historical data and real-time data streams from diverse sources, including IoT devices, social media, and customer interaction platforms. This allows insurers to incorporate a much broader range of information, providing a more holistic view of risks and customer behaviors. AI and machine learning allow these models to dynamically adjust to new data inputs, continually refining predictions and uncovering patterns that traditional methods might miss. For example, machine learning algorithms can identify subtle correlations between variables that would be invisible in rule-based systems.
With these advanced tools, insurers can make more proactive, data-driven decisions. This includes personalized pricing, real-time fraud detection, and predictive maintenance in areas like property and casualty insurance.
As customer data proliferates and coupled with the declining costs of computing power and data storage, companies are increasingly investing in data analytics to drive innovation. The emergence of powerful new analytics tools is enabling insurers to leverage this data in unprecedented ways, unlocking opportunities that were previously unimaginable. Early adopters are already reshaping their business models to tap into this potential and those who hesitate risk falling behind.
Topics: Data Security


